Before we go deep into machine learning poisoning, we need to know the basic machine learning concept.
As the words are self-explanatory, machine learning is basically the machine’s automation to learn and program itself into doing new tasks. For example, you use your smartphone for a lot of functions. You use a lot of web searches init. But you may have noticed that when you search for a specific item or thing, the ads section on the website starts to show you the ads related to your searches; that is called machine learning.
Now let’s take a deeper dive into it. Suppose a machine learning application is supposed to learn things from you and your surroundings, just like Siri, Cortana, and Alexa. You use this type of application in many places. You have your personal details in it. But what happens if the security in that new application is not that good and someone injects a viral code init, which can spin out your personal details to the hacker. This process is called machine learning poisoning.
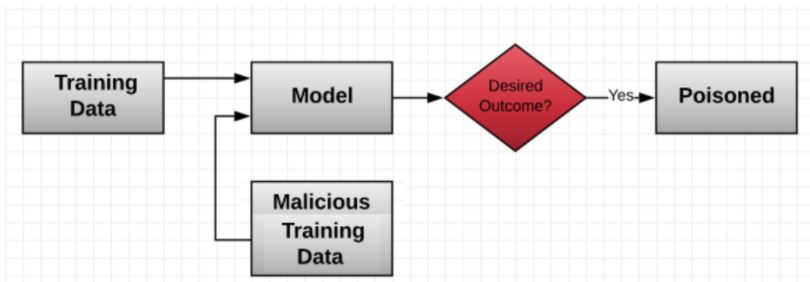
What is machine learning poisoning?
Machine learning poisoning is one of the most common techniques accustomed to strike Machine Learning systems. It defines attacks in which someone deliberately ‘poisons’ the teaching data used by the algorithms, which end up weakening or manipulating data.
Machine learning poisoning is a way to deliberately feeding machine learning algorithms insufficient data so that they can make wrong decisions and give unsuitable outcomes. The online survey is one example of machine learning poisoning, as it may answer the survey question so that it can sound sarcastic, which at times leaves the survey taker amused. However, in the same way, if many people engage in such activity, then the main reason for the survey will be changed, and if the survey taker assumes and take actions as per the result of the survey, so he will end up making some horrible decision.
How can the attackers poison the machine learning algorithm?
They are quite a few ways through which cybercriminals can manage machine learning poisoning, out of which a few of them are mentioned below.
- Poison through transfer learning:
Hackers can train an algorithm poison and then escalate it to a brand new machine learning (ML) algorithm with the transfer learning. This technique is very vulnerable as the poison data can be wiped out or go down by non-poisoning data.
- Data injection and data manipulation:
Data injection is when hackers inject the bad or corrupted data into the teaching data pool of the Machine Learning algorithm. Whereas data consumption needs more connection to the device training data, as it turns out the place where hackers alter the current data or information, the hackers can play with the labels as it shows cat but show the label as “DOG.”
- Logic corruption:
The most impressive and effective poisoning strike is known as logic corruption where the hacker poison and change the method of the learning of the algorithm. The results come out that the algorithm couldn’t able to learn in the right way or correctly.
What are the types of attacks?
There is various type of attacks or strikes that sum up like machine learning poisoning. The attack hacker will depend on various elements, which comprise the hacker or striker’s target and the amount of information and level of access they have to the Machine Learning system.
The majority of deadly attacks enter a sufficient amount of corrupted training data that the system started to give wrong or bias output. To put it another way, the machine learns inaccurate or wrong categories and prejudice.
A more advanced machine learning poisoning attack is the one that poisons the training data to generate a secret door but not to move borders. This means that evil or wrong data educates the system a vulnerability that the hacker can later use. Apart from this fault, there is no other weakness in the machine learning system.
What are the consequences of machine learning poisoning?
It is not easy to talk about the possible consequences in a familiar way because machine learning is utilized for the vast diversity of causes. Consequences from a strike against a machine learning algorithm used in reserve application data are very different from the consequences of a strike against the driverless car’s learning algorithm. In one situation, the striking result is data loss, and in the other situation, the striking result is the loss of human life.
The fascinating thing about machine learning poisoning is that there can be different stages or levels of poisoning. Algorithms can extend avidly, but the small number of bad or wrong data is not likely to affect the machine learning algorithm to enable it to make a wrong decision. There is some algorithm that is specifically designed to avoid deviation. However, a machine learning algorithm that is continuously fed with a stable amount of inaccurate data eventually started to act unexpectedly. Anyway, machine learning algorithms are made under the idea that the massive amount of data they receive is valid, and the decisions made by the algorithms depend on the data or information given to them.
Hope the readers would have more clarity after reading this piece. Any comments or suggestions are welcome as we strive to make technology easier for everyone.