The advancements in identifying the space objects has now taken a big giant leap with the help of the artificial intelligence and machine learning. Using these AI teams across the globe we are now able to take accurate and precise measurements of the deep space objects and also we are able to determine the nature and the spectra of the photons which are being emitted by the object in the night sky. A very recent example on how the AI is used efficiently can be seen from a team at Portuguese Institute of Astrophysics and Space Sciences where they have used the machine learning techniques for the recognition of the space objects. The interface which is being used is called SHEEP which has been used before to find the extraterrestrial lives on other planets. Like all other algorithms, SHEEP works just like another facial recognition software with similar data sets which is used to identify the difference between various daily objects and then separate or catalog them into categories.
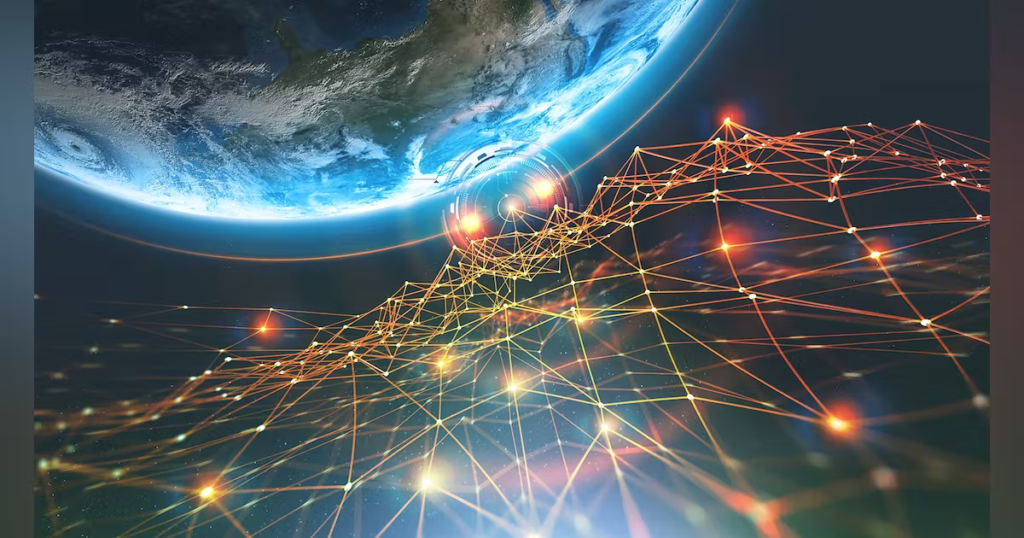
Works done on Space Exploration
Artificial intelligence will further enhance space exploration in the near future. Indeed, intelligent tools which analyse the data and predict the future events will be used in every aspect of space settlement. Automation and robotics have always played a significant and vital role in terms of space exploration, opening new frontiers and improvements since the beginning. The exploitation of AI contributes to creating independent and intelligent robots which are able to learn even while they are in orbit. In addition to this, the management of space traffic and the assistance of ground operators can also be supported using various AI techniques.
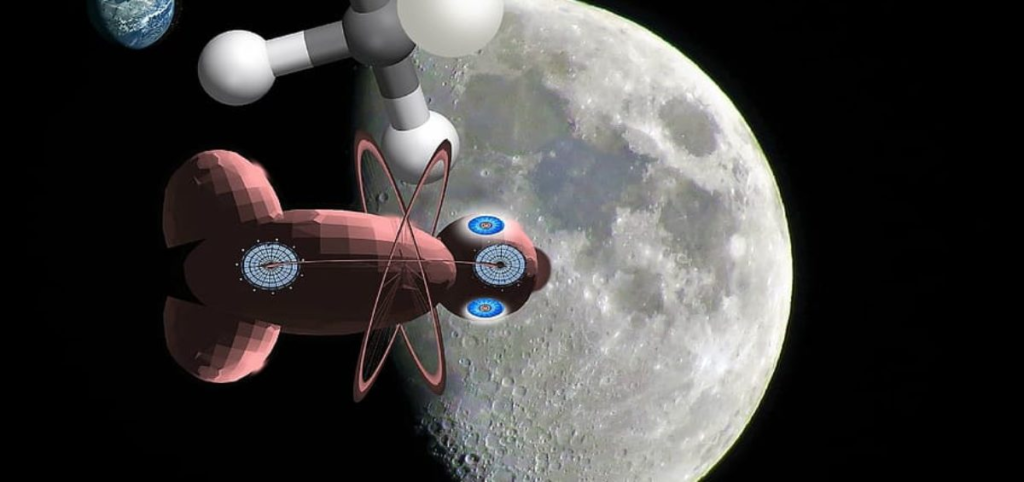
Improving the autonomous functions of space robots, spacecraft, and equipment is a need that could be achieved using the AI systems. They primarily focus on evolutionary optimization, tree searches, and machine learning using the deep learning and reinforcement learning, analysing the interesting use cases where these algorithms have even improved the performances of guidance and control of well-known problems. The use of swarm cooperative rovers is based on a set of shared information supporting the mission planned to reach a common target. In a cooperative, coevolutionary algorithm which is used to train a multi-robot system is presented. Any space exploration scenario presents us with several challenges that can be met jointly by both humans as well as swarm robots. Also, AI techniques play an important and crucial role in astrophysics and astronomy. AI searches the space in planetary studies and conducts real-time monitoring of the instruments and investigations of stellar clusters and non-stellar components of the Milky Way. Notably, as suggested, both deep learning and neural networks can automate the detection of astronomical bodies and sustain the future exploration missions.
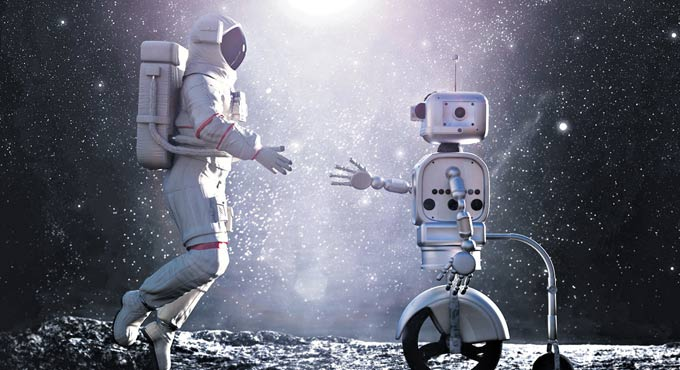
Works on Earth Observation
The survey has been done which reviews machine learning models used to predict and understand components of the Earth observation system and divides the addressed problems into four different categories:
(1) classification of the land cover types.
(2) Modelling of the land–atmosphere and ocean–atmosphere,
(3) detection of any signs of anomalies and extreme events, and
(4) causal discovery.
AI and Earth observations (EO) can meet the sustainable development goals (SDGs) and specifically contribute to them in a big way.
The major motivations of this particular approach concern the full use of real-time sensing of data of multiple satellites from a smart constellation and therefore the on-the-edge processing of data and the resulting benefits in terms of the imaging product value. AI algorithms can be considered for consuming the data at the source rather than consuming the data on the ground, lightening the down-link load, and allowing the development of some value-added applications in the space.
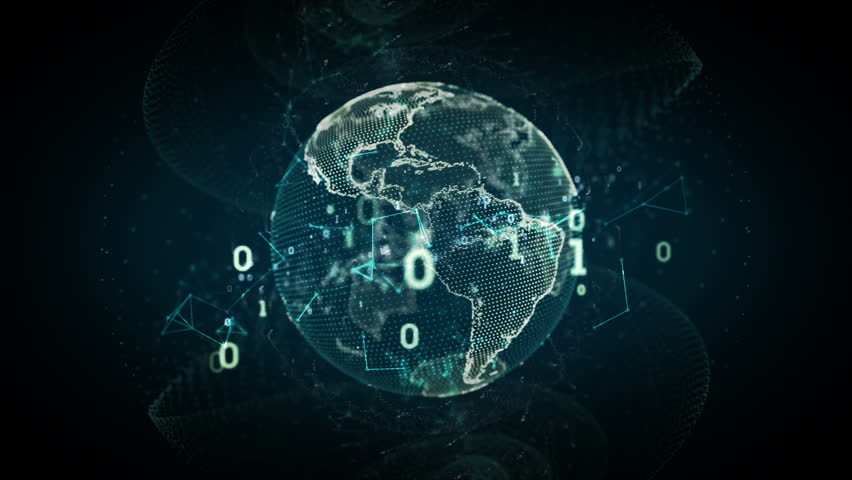
CONCLUSION
Deep learning in the recent days is being used widely by the researchers to respond or react to the space sector’s countless challenges. For example, neural networks are being used to improve the land use for the agriculture, mitigate the consequences of climate change, and monitor the water quality consistently. Another example of deep learning is the use of CNNs to assist the astronauts during their mission by facilitating the astronauts with the work and lives through an intelligent assistant. Furthermore, RNNs can also further improve telemetry analysis of space operations in order to temporally predict any anomalies on board.
AI is exploited in the contexts of many applications to perform various tasks in a human-like way, and it has an important position in the digital transformation of the society. As always, the research community will be having a important role in developing the new AI-based solutions for the most arduous or typical engineering challenges, and the survey papers have been published which solely focus on discussing such solutions in almost all the fields except the field of space applications. In this discussion we filled this gap by presenting a framework that surveys all the recent AI-based solutions used in the space applications and classified them based on the specific problem to which they are applied and the typology of the artificial intelligence approach which is used to solve that particular problem which in turn helps in developing a taxonomy of all the recently proposed solutions. The presented classification allows us to identify the most recent solutions which have been applied to solve some of the specific challenges and the most important challenges of space applications that AI-based approaches can help us in facing.