KNOWING ABOUT DATA WRANGLING
What is it DATA WRANGLING?
The process of cleaning, organising, and enriching raw data into a desired format for improved decision making in less time is known as data wrangling. Data wrangling is becoming more common in today’s top companies. Data has become more diversified and unstructured, necessitating more time spent selecting, cleaning, and organising data before doing a more comprehensive analysis. At the same time, with data informing almost every business decision, business users have less time to wait for prepared data from technical personnel. This needs a self-service paradigm, as well as a shift away from IT-led data preparation towards a more democratised self-service data preparation or data wrangling model. This self-service architecture with data wrangling tools enables analysts to work with more complex data faster, deliver more accurate results, and make better judgments. As a result of this capability, more companies are beginning to adopt data wrangling technologies to prepare data for analysis.
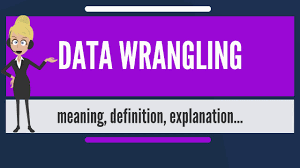
What will it do:
Wrangling is, in fact, just as important as the end results in the data analysis process. When done correctly, data wrangling provides insights into the nature of the data, allowing you to ask better questions of it. Iterative wrangling is more effective than one-time wrangling. Each step in the wrangling process reveals new possibilities for “re-wrangling” the data, all in the service of producing the most reliable final analysis. To ensure that the final dataset is reliable and accessible, each data project requires a unique strategy. Nonetheless, the method is usually informed by a number of procedures. Data wrangling steps or activities are what they’re called.
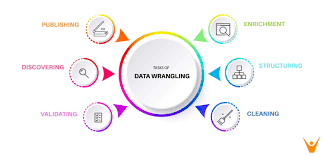
- Discovery: The term “discovery” refers to the act of becoming familiar with data so that you can imagine how you may use it. It’s similar to checking your refrigerator before cooking a dinner to see what items you have on hand. You may see trends or patterns in the data during discovery, as well as evident errors such as missing or incomplete values that need to be corrected. This is a crucial stage because it will inform all subsequent activities.
- Structuring: Data arrives in different shapes and sizes, thus structuring is required. For instance, you might have a transaction log with one or more objects linked with each entry (think shopping basket). To perform an inventory analysis, you’ll probably need to break down each transaction into distinct records for each item purchased. Alternatively, you may look at which products are frequently purchased together. In this scenario, it might be fair to broaden each transaction to include every pair of purchased items.
- Cleaning: You must first ensure that the data is clean before entering it into any analytic software systems. Cleaning data involves removing duplicates and null values, as well as relying on formatting to improve data quality. You should also standardise your data. This is where you’ll put everything in a column in the same order, such as “CA,” “Calif,” and “California.” Data cleaning is essential for data mapping and accuracy. You may build up rules to clean automatically with automation software that connects directly to systems. By automating this highly manual low-value operation, map data eliminates any guesswork and saves a significant amount of time.
- Enriching: You must assess whether you have all of the data required for the project on hand once you have a better understanding of your existing data and have turned it into a more useful condition. If not, you can enrich or supplement your data by adding values from other datasets. As a result, it’s critical to know what other data is available for use.
- Validating: The process of ensuring that your data is both consistent and of sufficient quality is known as data validation. You may identify flaws that need to be resolved during validation, or you may conclude that your data is ready to be examined. Validation is usually accomplished through a series of automated processes that necessitate programming.
- Publishing: You can post your data once it has been validated. This entails making it available for examination to others in your organisation. The manner in which you provide the information, such as a paper report or an electronic file, will be determined by your data and the aims of your organization. You must publish and distribute the data in order for an organisation to use it after the wrangling process is completed. This could include uploading the data to automation software or keeping the file in a location where the company knows it is ready to use. For future reference, it’s also a good idea to describe the processes taken and reasoning employed in the data wrangling process.
Why:
Quality data is entered into analytics or downstream processes for consolidation and cooperation when data is correctly wrangled. To shorten the data-to-insight process and assist quick decision-making, data wrangling is critical. Using data integration tools with automated features that clean and convert source data into a reused format as per the end needs, data wrangling may be organised into a consistent and repeatable routine. Data professionals spend about 73 percent of their time merely wrangling data, making it an essential part of the data processing process. By cleaning and arranging raw data into the desired format, data wrangling assists business users in making concrete, timely decisions. Data wrangling is becoming more frequent among top firms as data becomes more unstructured and diversified. You may do critical cross-data set analyses after converting data to a common format. Furthermore, Python data wrangling is frequent, as Python uses a variety of methods to wrangle data contained in various data sets.
Advantages :
Data confrontation is so advantageous in simple terms, since it is the only way to make raw data usable. Many times, customer information or financial information are available in a practical business setting in various departments. This information can sometimes be stored on different computers across various spreadsheets, including legacy systems for the duplication of information, incorrect data and data not found to be used. It is best to use all data in a centralised location to create a whole picture of what is happening within a company. This is just one way for data automation tools to help process data disputation.
When it comes to data wrangling, it’s important to be able to piece together raw data while also comprehending the data’s business context. Good data wranglers will be able to analyse, clean, and transform data into useful insights in this manner. Automation technologies also decrease errors, map out processes to reduce crucial man reliance, eliminate low-value manual chores so that employees can focus on the high-value tasks that matter, and save employees time so that they can deliver more and better insights to the business.
Conclusion:
Data wrangling is an essential part of every company’s operations. It’s used to turn unstructured data into useful information. This critical process has been carried out manually, but it does not have to be. Your data analyst will be slowed down by manual data wrangling since he or she will be changing data and filling in gaps rather than performing analysis. It’s crucial to remember that data wrangling, especially when done manually, may be time-consuming and resource-intensive. Many companies have policies and best practices in place to help employees streamline the data clean up process, such as requiring data to include specific information or be in a specified format before being uploaded to a database.
Problems may occur at any point in the network, if we feel stuck any point, we can solve it simply by taking an expert help in fixing the problems in your process or your system our customer friendly team “computer repair on site” is always as a solution for all the software and hardware problems. access their website is also very easy, to get an instant help click here.
Consider using a data automation solution if you get any issue with the data we can take the help of the professionals like the team of “BENCHMARK IT SERVICES” here we not only get the solutions for the data issues but also they help us to aid in data wrangling, data management, and automated analytics to improve your decision-making process by providing more precise and accurate insights, as well as real-time analytics and reports. you can access their customer friendly website here.